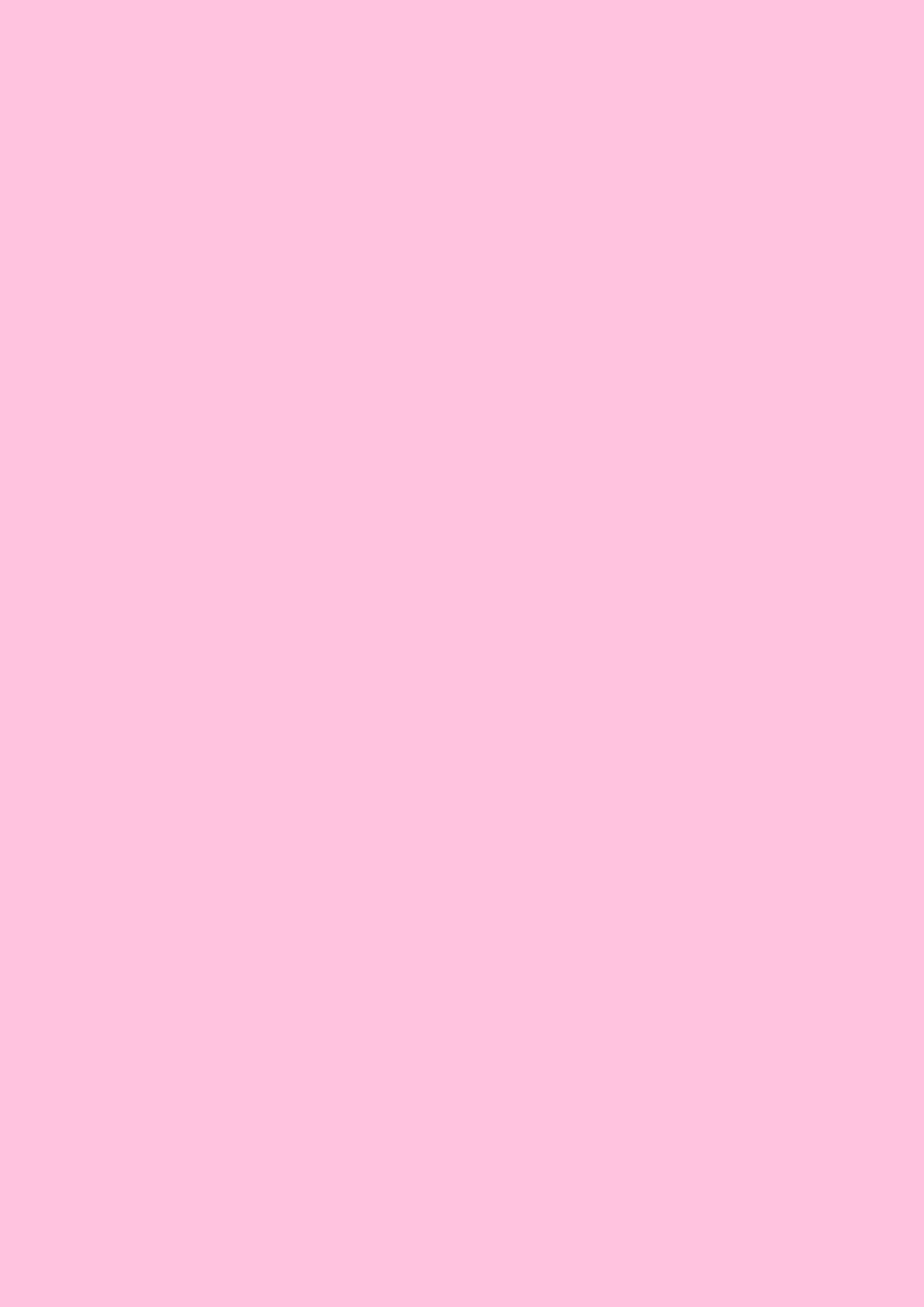
Artificial Intelligence
Cultural stereotypes can be found in artificial intelligence (AI) technologies already in widespread use. For example, when Londa Schiebinger, a professor at Stanford University, used translation software to translate a newspaper interview with her from Spanish into English, both Google Translate and Systran repeatedly used male pronouns to refer to her, despite the presence of clearly gendered terms like ‘ ’ (female professor). Google Translate will also convert Turkish sentences with gender-neutral pronouns into English stereotypes. ‘O ,’ which means ‘S/he is a doctor’ is translated into English as ‘He is a doctor’, while ‘O (which means ‘S/he is a nurse’) is rendered ‘She is a nurse’. Researchers have found the same behaviour for translations into English from Finnish, Estonian, Hungarian and Persian.
The good news is that we now have this data – but whether or not coders will use it to fix their male-biased algorithms remains to be seen. We have to hope that they will, because machines aren’t just reflecting our biases. Sometimes they are amplifying them – and by a significant amount.
​
James Zou, assistant professor of biomedical science at Stanford, explains why this matters. He gives the example of someone searching for ‘computer programmer’ on a program trained on a dataset that associates that term more closely with a man than a woman. The algorithm could deem a male programmer’s website more relevant than a female programmer’s – ‘even if the two websites are identical except for the names and gender pronouns’. So a male-biased algorithm trained on corpora marked by a gender data gap could literally do a woman out of a job.
​
But web search is only scraping the surface of how algorithms are already guiding decision-making. According to the 72% of US CVs never reach human eyes,45 and robots are already involved in the interview process with their algorithms trained on the posture, facial expressions and vocal tone of ‘top-performing employees’.46 Which sounds great – until you start thinking about the potential data gaps: did the coders ensure that these top-performing employees were gender and ethnically diverse and, if not, does the algorithm account for this? Has the algorithm been trained to account for socialised gender differences in tone and facial expression? We simply don’t know, because the companies developing these products don’t share their algorithms – but let’s face it, based on the available evidence, it seems unlikely.
​